Machine Learning (ML) has found applications in almost every industry, but the way it’s accelerating the pace of discovery and advancement in healthcare is commendable. The healthcare sector remains one of the largest generators and consumers of data, generating over 2,000 exabytes in 2020 alone.
Apart from managing and securing data, hospitals need to provide top-notch care and treatment to create an optimal experience for patients. Let’s take a look at the uses of ML in healthcare and outline how you can leverage ML to improve healthcare delivery.
Machine Learning has emerged as a 360-degree solution that helps healthcare providers tackle all these challenges and many more. It enables better data management and helps providers make informed treatment decisions.
Digital Revolution in the Healthcare Sector
The digital revolution has influenced every industry, and healthcare is no different. In fact, many consider tech advancements in the medical and pharmacy sectors as the most prominent developments of the 21st century.
The health tech industry is booming and is projected to reach US$280 billion by the end of 2021, growing at a CAGR of 15.9%. However, some people still don’t fully understand the role of technology in healthcare.
So, let’s start by defining digital health first.
Digital health is connecting and empowering people to manage their health and wellness by providing accessible, on-demand, interoperable, integrated, and flexible medical services and support.
In a nutshell, digital health is all about allowing people to take advantage of healthcare services through digital technologies. The technologies used to facilitate digital health are collectively called healthcare technology.
A significant example of digital health is telehealth, which allows healthcare providers to administer non-clinical services online through telecommunications.
Some other aspects of digital healthcare are:
- Wearable medical devices for remote health monitoring
- Robot-assisted surgery (RAS) for increased accuracy and efficiency in surgery
- 3D bioprinting to automate the production of medical devices, transplants, and prosthetics
- Virtual and Augmented reality to treat stress disorders
- Data Analysis, Big Data, and Artificial Intelligence (AI) for data collection and analysis
- Electronic health records in place of physical registers
- Custom QR code solutions to store, share and track electronic data
- Blockchain for secure the handling of electronic health records (EHR)
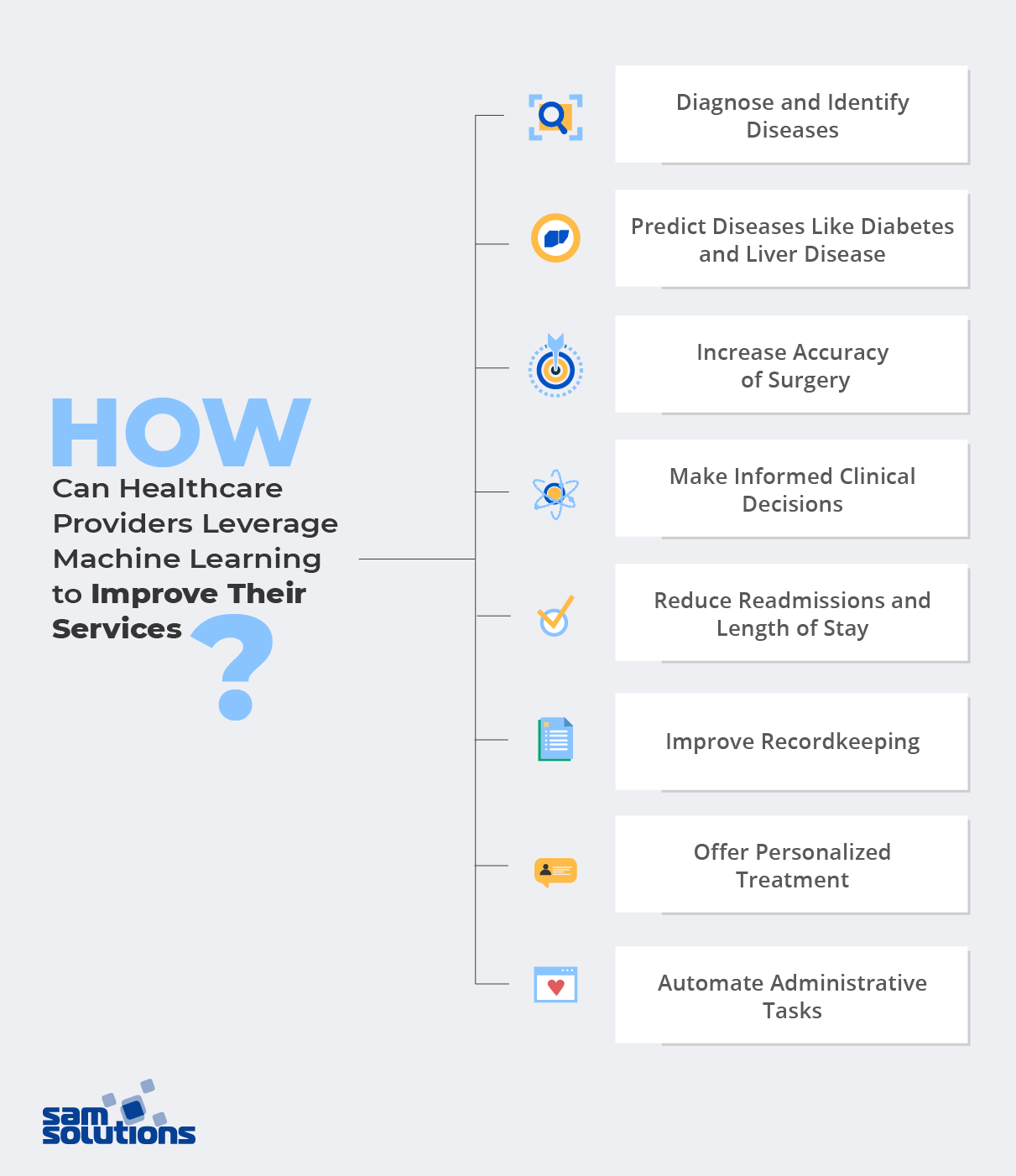
ML Is Reshaping Healthcare
Machine Learning (ML) is a subset of AI that enables machines to learn from the data fed to them without explicit programming. The machines use underlying algorithms to “learn” from computational data and offer predictable outputs. This paves the way for several applications, such as the development of clinical care guidelines, clinical decision support, and automated medical billing.
Many ML scientists have developed algorithms to predict certain medical conditions in patients. Radiologists can also benefit from ML. Deep Learning, an application of ML, can assist radiologists in reviewing radiographs, MRI scans, and CT scans and make accurate decisions. Recordkeeping, data integrity, and predictive analytics are other areas where ML plays an instrumental role.
How Can Healthcare Providers Leverage Machine Learning to Improve Their Services?
Machine Learning is the technology of the future. It can help hospitals, clinics, labs, and other medical facilities make their operations faster and more accurate. But regardless of how revolutionary the technology is, you can’t derive value from it if you don’t know how to implement it.
Therefore, healthcare providers need to understand the applications of ML and how they can implement ML in their day-to-day processes. Here are ten ways you can leverage ML to improve service delivery and pave the way for a healthier future.
Diagnose and Identify Diseases
One of the key applications of ML in healthcare is disease diagnosis and identification, especially for hard-to-diagnose diseases. For instance, ML can help diagnose tumors and cancers in early stages or genetic diseases beyond traditional diagnostic methods. IBM Watson Genomics, a part of IBM Research, integrated genome-based tumor sequencing with cognitive computing to diagnose early-stage tumors.
Healthcare providers can also use ML to enhance medical image diagnosis. Some diseases can only be diagnosed by image analysis. However, evaluating imaging results with the naked eye is prone to error. You can use tools like InnerEye by Microsoft to scan thousands of medical images per hour with top-notch accuracy.
You can also use ML-based algorithms to improve radiotherapy outcomes. Medical imaging has numerous minute details that are difficult for a radiologist to see. ML algorithms can analyze various imaging samples to improve diagnostic results.
Predict Diseases Like Diabetes and Liver Disease
Machine Learning does a great job at diagnosing early-stage conditions. However, certain diseases are difficult to cure after symptoms are developed. ML helps predict these diseases, allowing healthcare providers to better manage high-risk patients and improve prognosis.
Diabetes is a common illness that affects around 500 million people, or 10% of the world population. Uncontrolled diabetes can damage a person’s nerves, heart, and kidneys and lead to other serious illnesses. Most people are diagnosed with type-2 diabetes only after they experience symptoms. Unfortunately, by the time symptoms appear, the disease will usually have already developed to an advanced stage.
ML lets you identify people in prediabetic condition. If they receive treatment at that stage, the advancement of the disease can be prevented.
The same goes for other deadly diseases like liver disease and skin cancer. Liver conditions like chronic hepatitis, cirrhosis and liver cancer are difficult to predict, and once they develop, they’re almost impossible to cure. You can use datasets like the Liver Disorders Dataset to predict these conditions before they begin to advance.
Increase Accuracy of Surgery
Some surgical procedures like craniectomy, aortic dissection repair, and bladder cystectomy are increasingly complex and carry significant risks. They require a high level of precision, and a minor human error can lead to life-threatening complications. Robotic surgery has solved this hurdle to a large extent.
Surgeons can use robots like the Da Vinci robot to perform surgeries with precision while focusing on fine details. Integrating ML with surgical robots can take robotic surgery to the next level. For instance, you can use ML algorithms to incorporate previous surgical data and metrics to enhance the precision of surgical robots.
A good example of ML-robotics integration is Mazor Robotics. The company combines AI/ML with robotics to minimize the invasiveness of surgical procedures associated with complex body parts like the liver and spine.
Make Informed Clinical Decisions
Natural Language Processing (NLP), an application of ML, allows you to collect unstructured data from health records and medical imaging and turn it into editable documents with actionable insights. This helps doctors see a clear picture and make informed clinical decisions.
Let’s demonstrate this concept with an example. Normally, patients make an appointment, consult a doctor, and describe the problems they have. Based on the information provided by the patient, the doctor predicts the underlying conditions and begins treatment. NLP can speed up this process significantly.
When making appointments, patients can mention all the symptoms/problems they have, and the system can use NLP and ML to propose a treatment plan and send it to the doctor. So, by the time the patient meets the doctor, the doctor will already know about the patient’s condition and the best possible treatment. The doctor’s role will only be to approve the treatment and make changes to it, if any.
Doing so has two benefits. Firstly, the process becomes faster and more efficient. This way, clinics can attend to more patients in less time. Secondly, it increases decision-making accuracy. When doctors attend to patients, they may miss out on some symptoms and possible causes of disease. Machine learning helps them eliminate such errors.
Reduce Readmissions and Length of Stay
The 30-day readmission rate is 18.3% among Medicare patients and 11.8% among uninsured patients. Readmissions are a major challenge for healthcare providers and can be significantly costly. ML-based tools enable medical institutions to improve care delivery by predicting which patients are likely to be readmitted and how to prevent this from happening.
The length of stay (the average number of days a patient stays in hospital after being admitted) is another area of concern healthcare providers need to address. Since ML-based tools help improve the quality of care, they can also assist hospitals in reducing the average length of stay.
Improve Recordkeeping
Storing and maintaining health records has always been a challenge for healthcare providers, but not anymore. You can use ML-based OCR recognition techniques to classify health records.
A major application of ML in recordkeeping is the concept of smart health records. ML-based tools like handwriting recognition technology by MATLAB and Cloud Vision API by Google enable the maintenance of up-to-date health records.
Almost all healthcare providers store patient information online. While this adds to efficiency, it also raises cybersecurity concerns. Patient records have always been a lucrative target for hackers, making patient privacy a major concern for medical institutions.
Machine learning tools can play a vital role in improving the security of patient records. These tools can leverage behavioral analytics to send clinics alerts if there’s an attempted data breach. This way, healthcare providers can act promptly if a security issue arises.
Offer Personalized Treatment
Personalization is no longer limited to brands offering services to their customers. Medical facilities can also provide personalized treatments to patients using ML and predictive analytics. Presently, most doctors take a one-size-fits-all approach by administering the same treatment to all patients exhibiting a particular set of symptoms.
ML-based personalized medicine enables doctors to suggest multiple treatments to patients based on their symptoms and past medical history.
Another breakthrough application of ML in healthcare is behavioral modification. Research shows that behavioral and lifestyle changes affect a person’s health. Therefore, it becomes essential for doctors to monitor the behavioral and lifestyle characteristics of each patient. You can use tools like Somatix to monitor your patients’ lifestyles in a passive and non-invasive way.
Automate Administrative Tasks
Lastly, Machine Learning can enhance operational efficiency in healthcare settings. Research shows that 83% of physician burnout occurs due to the lack of proper organization, boiling down to excessive administrative tasks. This mainly includes reviewing and updating health records.
ML-based tools can solve this problem. NLP tools can capture vocal phrases and turn them into accurate text documents. Therefore, a doctor can dictate points to the EHR, and the built-in NLP tool can transcribe it into text. As a result, the doctor can spend less time on administrative tasks and more time with patients.
Over to You: How to Get Started?
ML is an essential technology for healthcare providers. However, many hospitals have long relied on traditional operational methods. A move to digital, ML-enabled models can be challenging.
Hospitals need to start small by developing a team of professional data scientists, also known as hospital analysts. Work closely with them to evaluate your resources and how you can incorporate ML into your day-to-day processes. Once you establish the benefits of ML for your healthcare facility, you can scale its uses.
It’s a groundbreaking idea! Robotic surgery and machine learning in healthcare will shape our future. Robots that use artificial intelligence are less likely to make mistakes and express any bias towards patients.
My doctor uses some kind of automation software, as far as I can judge. I don’t know the exact name, but it helps her find information about my previous visits and analyses quickly.
Why don’t all hospitals and medical centers use the latest ML technologies? Recently I had an unpleasant experience when I had to wait in a long queue to schedule an appointment.
I faced a lot of issues when I visited my doctor last month. Evidently, they didn’t use any automation, and that is a pity. It took so long to finally find my documents.
Thank you for the article. I think healthcare providers should pay more attention to new technologies. It would be wonderful to have all of your medical records in one place.